
DATA MINING TECHNIQUES. What is data mining? by Tanmay Terkhedkar
The process of data mining involves using tools and techniques to extract and effectively utilize data. The following two are among the most popular set of tools and techniques for data mining: R-language: It is an open-source tool used for graphics and statistical computing. It has various classical statistical tests, classification, graphical.
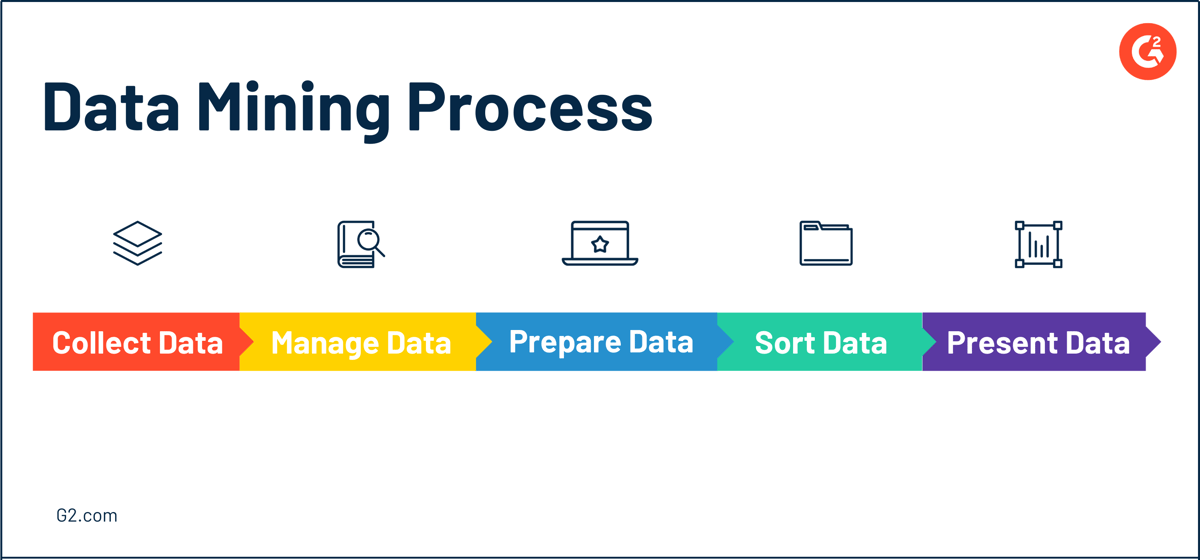
Data Mining Uncover the Valuable Business Insights You Need
The cross-industry standard process for data mining (CRISP-DM) is a guide to help start the data mining process. There are six phases for data mining: business understanding, data understanding, data preparation, modeling, evaluation, and deployment. The 6 CRISP-DM phases Business Understanding
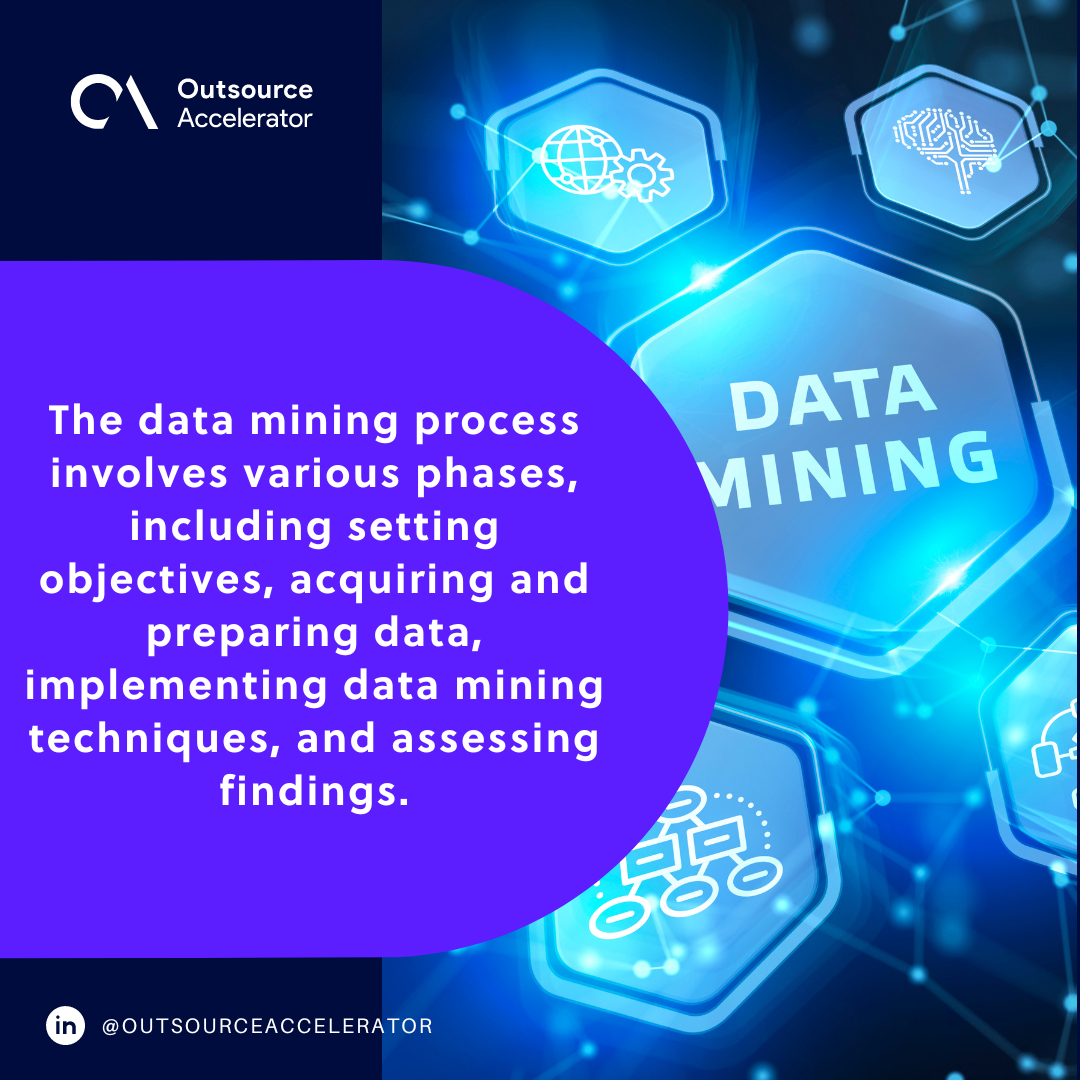
Data mining Outsourcing Glossary Outsource Accelerator
The data mining process starts with prior knowledge and ends with posterior knowledge, which is the incremental insight gained about the business via data through the process. As with any quantitative analysis, the data mining process can point out spurious irrelevant patterns from the data set. Not all discovered patterns leads to knowledge.
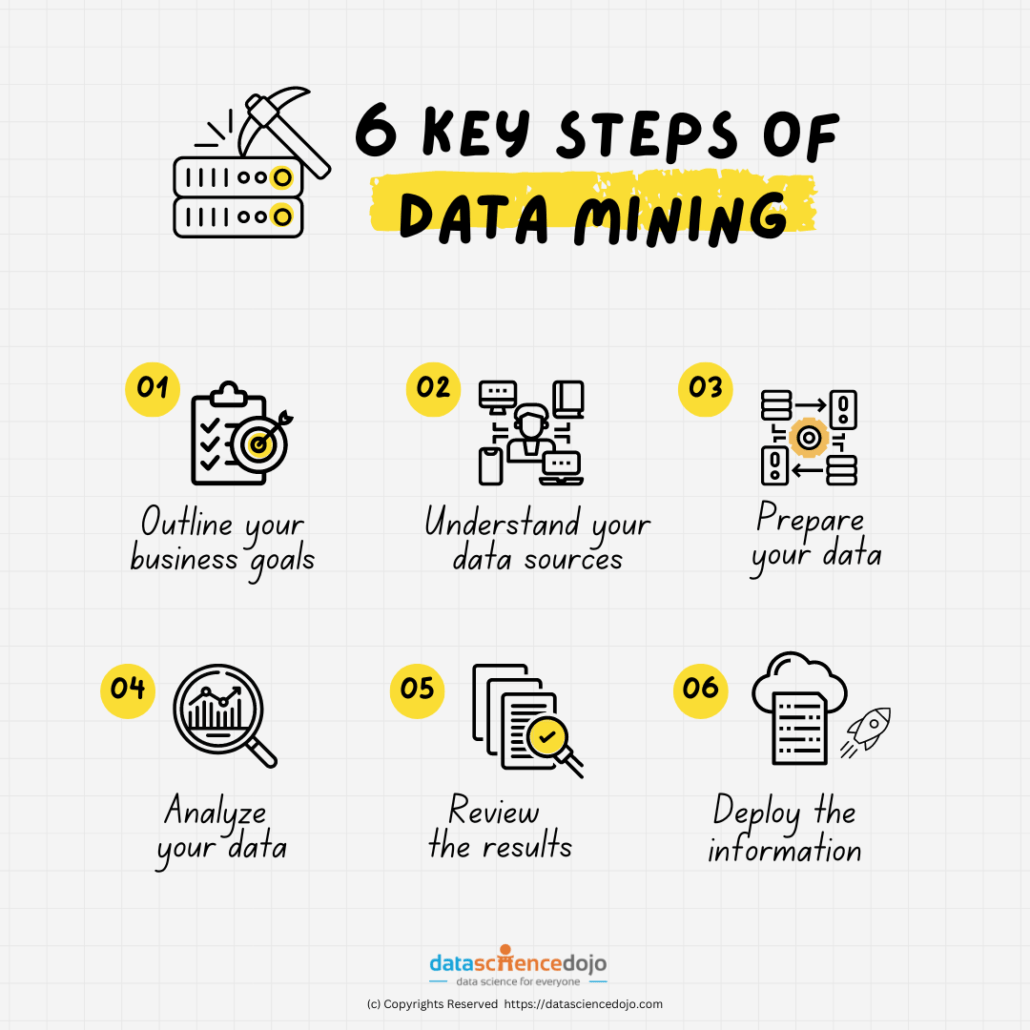
Sneak peek into data mining process Data Science Dojo
Data mining is a process used by companies to turn raw data into useful information. By using software to look for patterns in large batches of data, businesses can learn more about their.
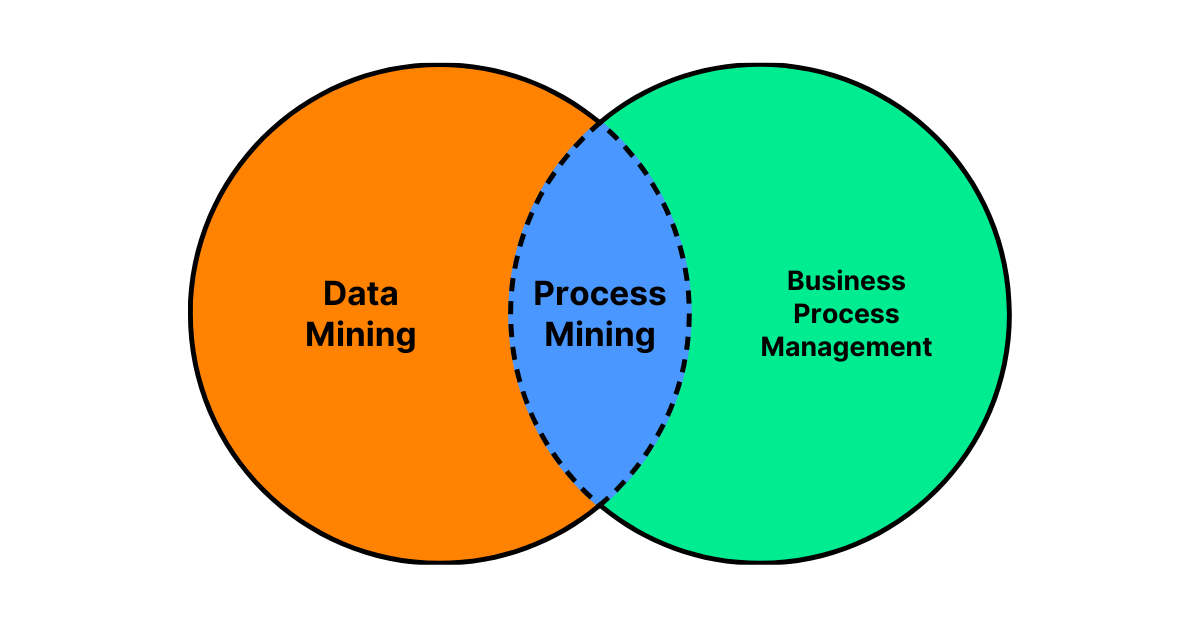
Process Mining vs Data Mining Workfellow
Data mining, also known as knowledge discovery in data (KDD), is the process of uncovering patterns and other valuable information from large data sets. Given the evolution of data warehousing technology and the growth of big data, adoption of data mining techniques has rapidly accelerated over the last couple of decades, assisting companies by.

The data mining process framework Download Scientific Diagram
Data mining is the process of finding patterns in data. The beauty of data mining is that it helps to answer questions we didn't know to ask by proactively identifying non-intuitive data patterns through algorithms (e.g., consumers who buy peanut butter are more likely to buy paper towels). However, the interpretation of these insights and.

Data Mining Steps Digital Transformation for Professionals
The overall goal of data mining process is to extract information from a data set and transform it into an understandable structure for further use. It is also defined as extraction of interesting (non-trivial, implicit, previously unknown and potentially useful) patterns or knowledge from a huge amount of data. Data mining is a rapidly growing.

Data mining Process Download Scientific Diagram
Here are the 7 key steps in the data mining process -. 1. Data Cleaning. Teams need to first clean all process data so it aligns with the industry standard. Dirty or incomplete data leads to poor insights and system failures that cost time and money. Engineers will remove all unclean data from the organization's acquired data.

6 essential steps to the data mining process
The illustrative definition of data mining. This process is essential in transforming large volumes of raw data — structured, unstructured, or semi-structured — into valuable, actionable knowledge. Brief data mining history. Data mining emerged as a distinct field in the 1990s, but you can trace its conceptual roots back to the mid-20th century.
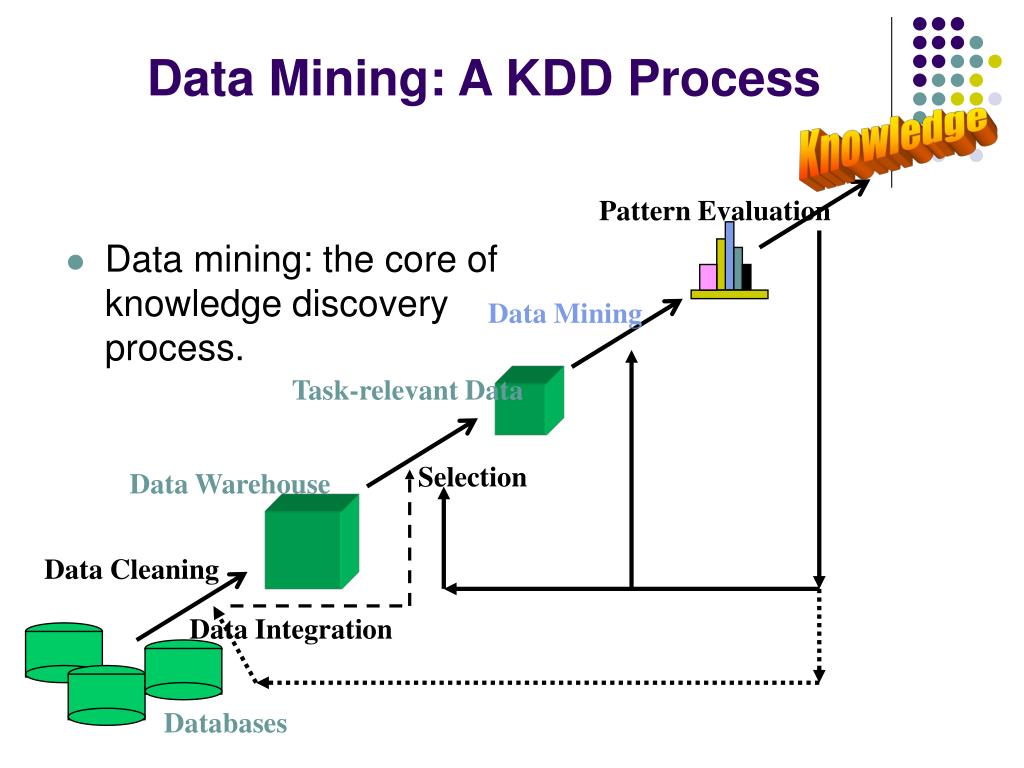
PPT Data Mining A KDD Process PowerPoint Presentation, free download
Data mining is the process of understanding data through cleaning raw data, finding patterns, creating models, and testing those models. It includes statistics, machine learning, and database systems. Data mining often includes multiple data projects, so it's easy to confuse it with analytics, data governance, and other data processes..
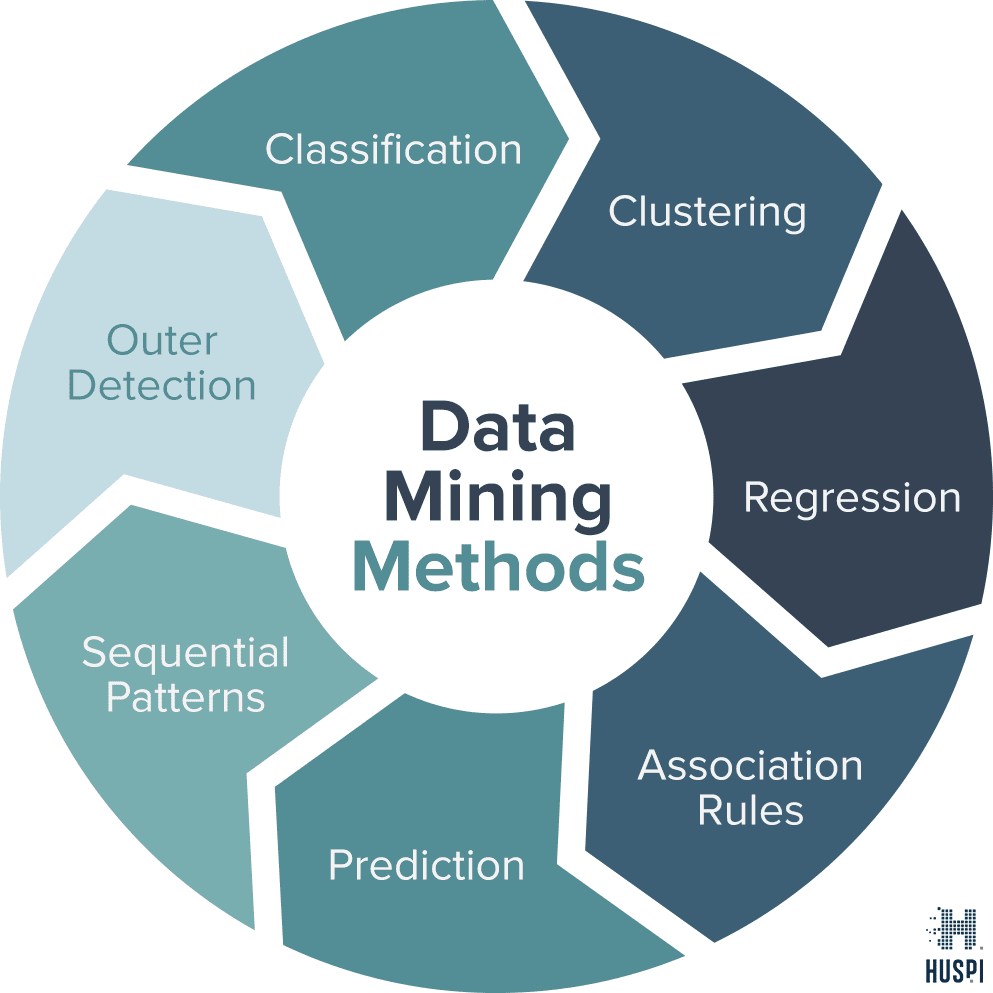
Data Mining How To A Brief Guide to Technology HUSPI
What Is Data Mining? Data Mining is a process of discovering interesting patterns and knowledge from large amounts of data. The data sources can include databases, data warehouses, the web, and other information repositories or data that are streamed into the system dynamically.
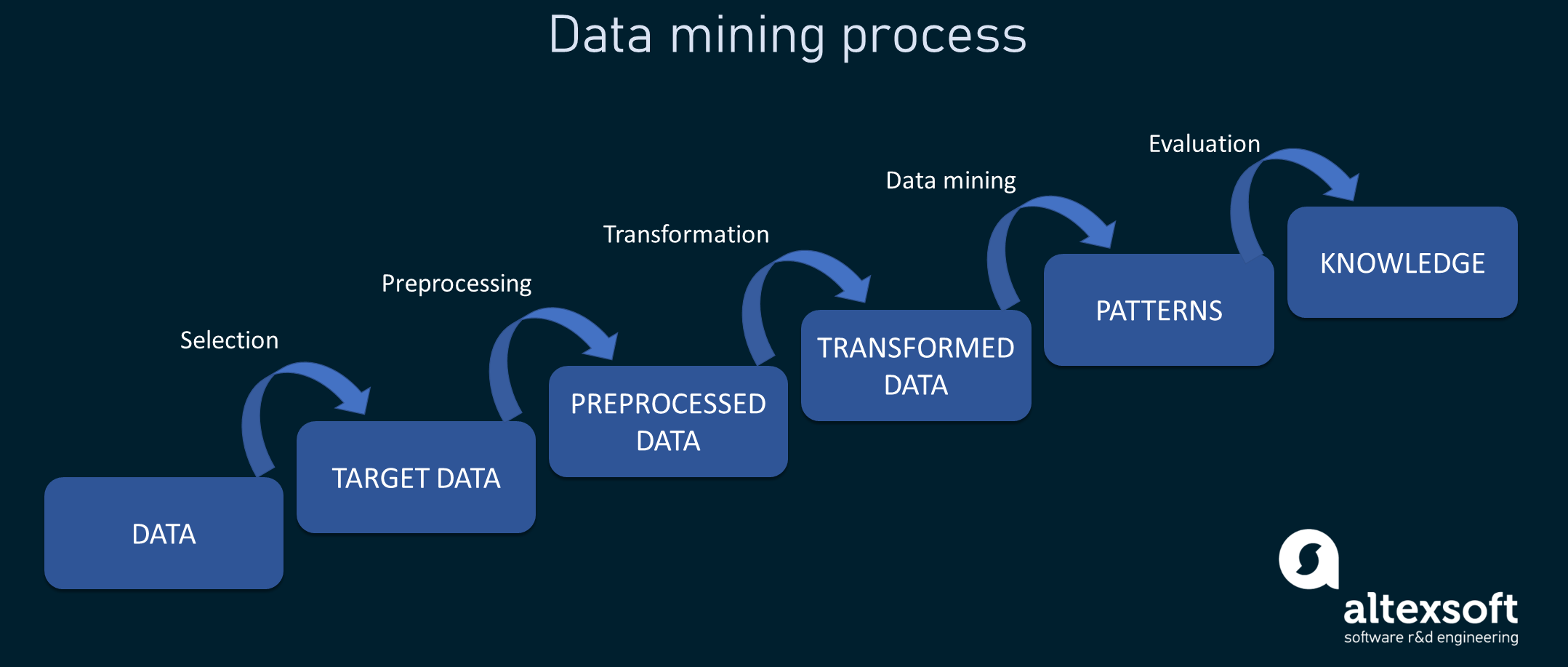
Data Science, AI, ML, Deep Learning, and Data Mining AltexSoft
Data mining is a systematic process of discovering previously unknown findings that hide within large datasets. The data mining process generally involves six main phases:Business understanding (Problem Statement), Data understanding,Data preparation,Data analysis,Evaluation,DeploymentIn each stage useful insights are gathered to support the development of an effective data mining strategy.
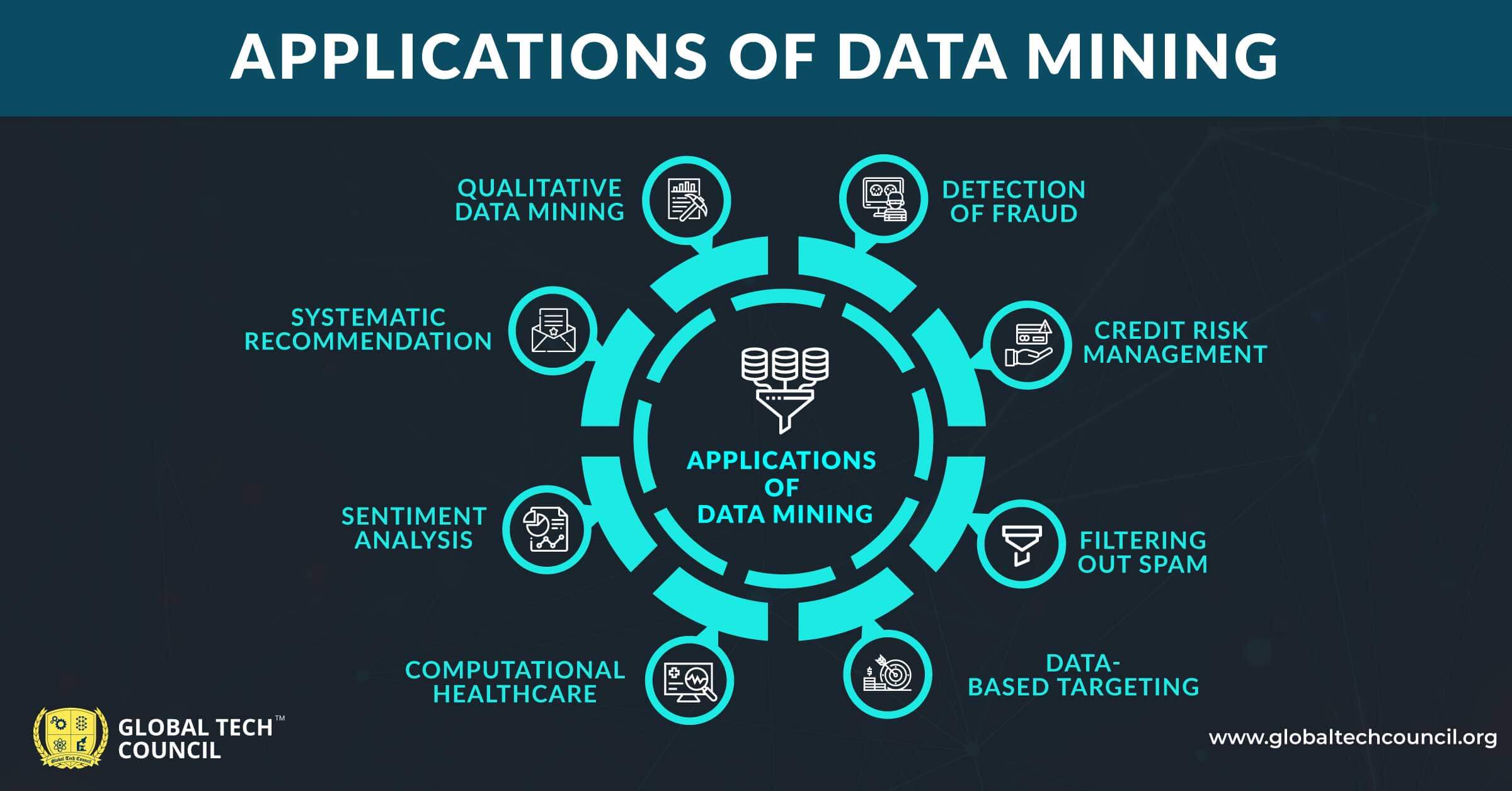
The Ultimate Guide to Understand Data Mining & Machine Learning
Data mining follows an industry-proven process known as CRISP-DM. The Cross-Industry Standard Process for Data Mining is a six-step approach that begins with defining a business objective and ends with deploying the completed data project. Step 1: Business Understanding. Step 2: Data Understanding.

Evolution of data mining Source Download Scientific Diagram
Data mining is the process of extracting knowledge or insights from large amounts of data using various statistical and computational techniques. The data can be structured, semi-structured or unstructured, and can be stored in various forms such as databases, data warehouses, and data lakes. The primary goal of data mining is to discover.
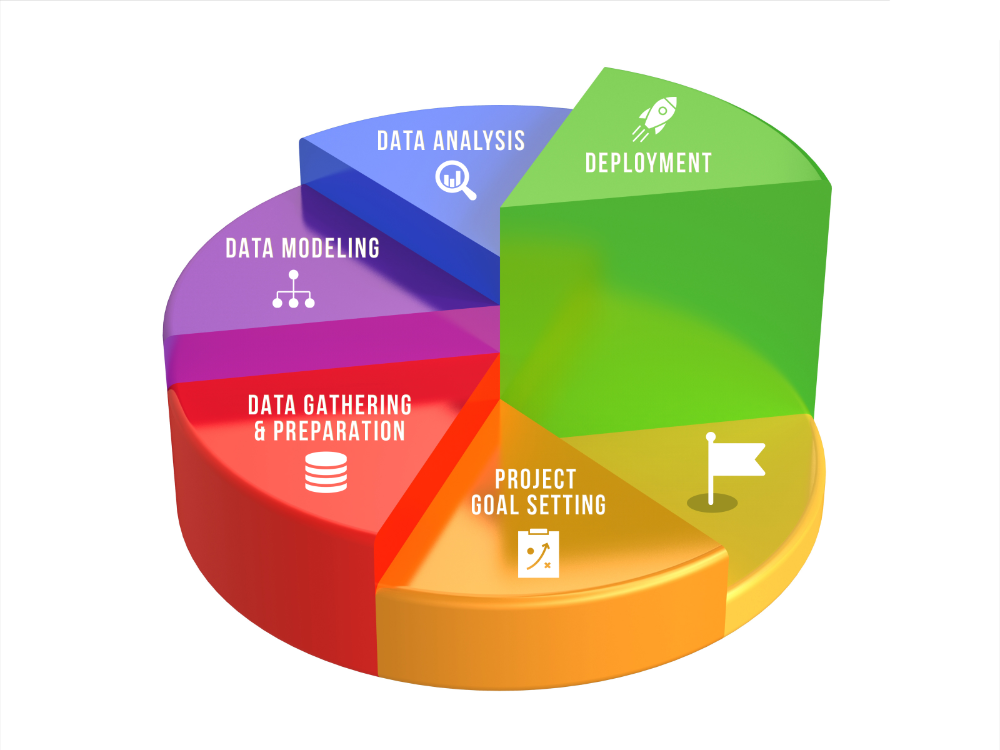
5 Data Mining Steps Hey DAN Voice to CRM
Data mining is the process of extracting and discovering patterns in large data sets involving methods at the intersection of machine learning, statistics, and database systems. Data mining is an interdisciplinary subfield of computer science and statistics with an overall goal of extracting information (with intelligent methods) from a data set and transforming the information into a.
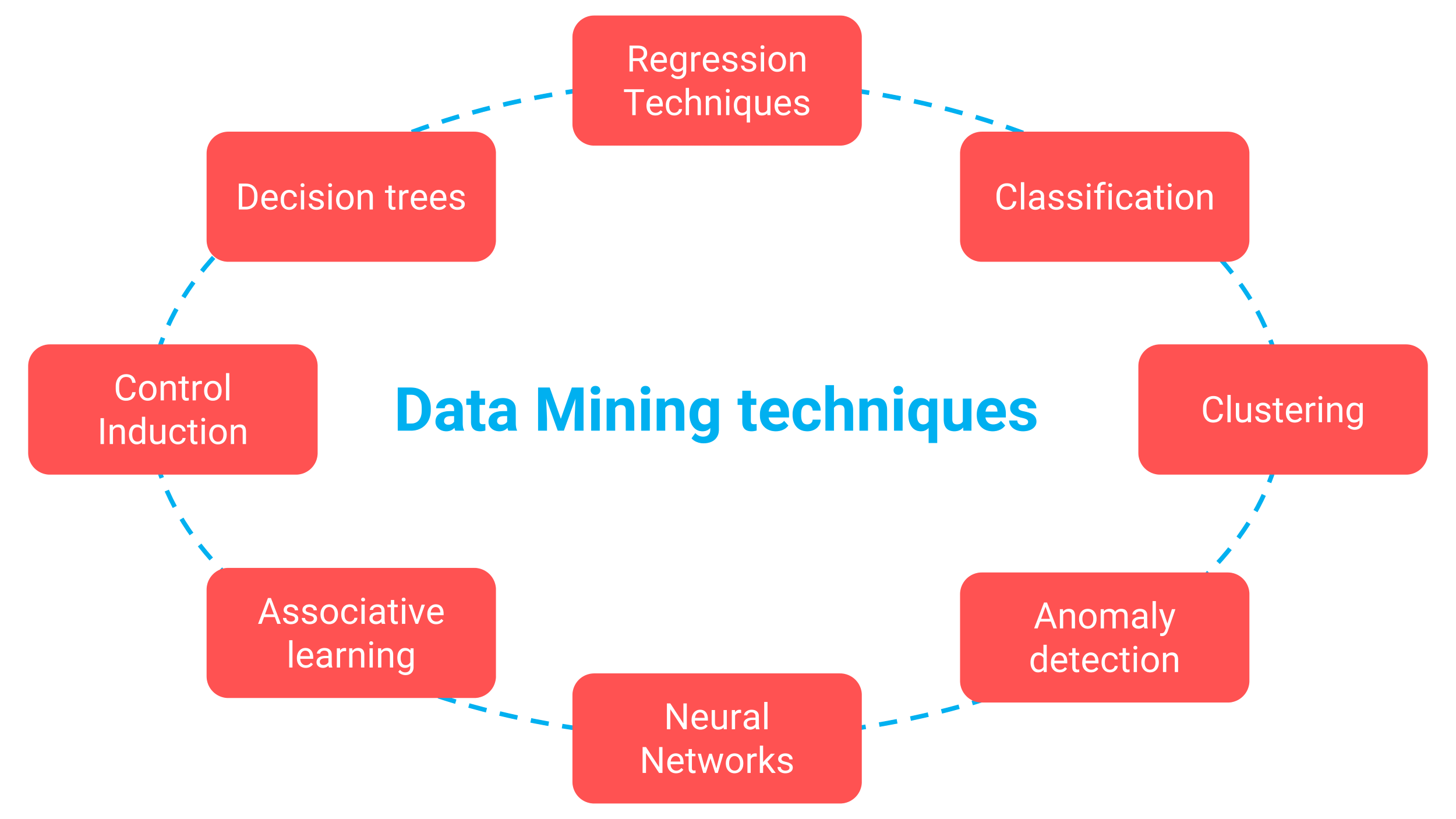
What is Data Mining? Give meaning to data mining in 6 steps
Data Mining Process In 5 Steps. The data mining process consists of five steps. Learning more about each step of the process provides a clearer understanding of how data mining works. Collection. Data is collected, organized, and loaded into a data warehouse. The data is stored and managed either on in-house servers or in the cloud. Understanding.